I was asked twice, in recent comments, for reference to a post defending my view that severe efficacy of the Covid vaccines is real (in older adults, not in kids). My answer was that I have never made such a post: Although I have said that severe efficacy is real a million times, I simultaneously do not consider this a view that requires explanation: It is a trivial observation.
For comparison, I have somewhat elaborate reasons for disputing the weight of evidence regarding “Long Covid efficacy” — the studies that show any such efficacy simply mix pre- and post-Omicron infection outcomes together, creating a “the cake is a lie” promise of effect. I have no such qualms with the evidence regarding severe efficacy.
Still, here is the post defending the view. Note that producing this post was not fun; it amounted to “homework.”
Summary:
When per-infection hospitalization rates are compared (apples to apples before or after Omicron), about five unvaccinated individuals are hospitalized for every vaccinated individual of the same age. If 100 older adults with a positive test were compared in the Delta era, 5 - 20 unvaccinated would wind up hospitalized, compared to about 1 - 4 vaccinated, depending on the age group and frequency of testing. (Of course, this is a smaller reduction in the amount of unvaccinated who aren’t hospitalized; 80 - 95 vs. 96 to 99, not even counting all those who don’t test.)
This finding — a ratio in the neighborhood 1/2 to 1/5 in favor of the (older, adult) vaccinated — was repeated over and over, in multiple regions in 2021. (After 2021, the unvaccinated almost all have natural immunity, and having “paid their dues” for it are now better off than the remaining, uninfected vaccinated.)
To dispute severe efficacy
Requires either 1) Believing that this divergence was the product of statistical manipulation, or 2) That it was a product of user bias, or 3) Not referring to the numbers directly at all, but merely making some biological argument which purports to determine the “true” numbers absent or in contradiction to evidence.
Statistical manipulation is not convincing given the universal replication of the similar per-infection ratios of hospitalization; user bias is not convincing in light of the scale of the ratio and realistic math regarding who got the vaccine; the biological arguments are all attempts to pencil-push a paradox in which “some immunity is worse than none.” (Actually, such a paradox may be valid for younger age groups, given the rarity of severe outcomes, but isn’t compelling when it comes to severe efficacy in older adults.)
Implications of severe efficacy
This, again:
I recycle the above (arbitrarily scaled overlap-having) diagram from my recent critique of Vaccine Nihilism, to open this post with the following question: What does believing or knowing the Covid vaccines reduce severe disease mean? What implications follow, regarding the use of the same vaccines?
For a comparative example, bicycle helmets maybe reduce harms from accidents — and yet whether they make riders safer is the topic of a decades-long debate, in which it has long been speculated that helmets promote unsafe riding behaviors, resulting in a wash or a net negative.1
This isn’t my stance with the Covid vaccines — rather, they are associated with safer behaviors — but illustrates that mere “working-ness” doesn’t establish whether a protective tool is worth using individually, or promoting to the lay public, or, obviously, mandating.
So, I believe the Covid vaccines work (reduce severe disease even in the case of having an infection), but should not be used or promoted to the lay public.
This is the same as my stance on masks and lockdowns, and the reasoning is the same:
Societies should not be worried about random new viruses that only rarely cause healthy younger people to go to the hospital. Even if such things are “bad,” there is an intrinsic, and low, limit to the bad, but unavoidable things that people can worry about without dismantling social function. For comparison: Rain and snow are rather detrimental; they destroy and kill; they cancel plans; but we didn’t used to scream at each other online about not taking rain and snow seriously enough. Now, for SARS-CoV-2: What happens if there is another new little virus tomorrow? Do we start all over again? I am only looking ahead — if it doesn’t make sense to do something over and over, then it doesn’t make sense to do it “just this one time.”2 Life would have carried on without the Covid vaccines.
Masks, lockdowns,3 and the Covid vaccines, because they are overkill in precisely the sense given above, induce fear among people at low risk — the younger adult PMC who is “too online,” precisely the individual responsible for lockdowns in the first place. They lead to screaming hysterically about something that we could have just lived with (like rain and snow).
Relatedly, because those tools suggest the bad thing in question is avoidable, they justify increased governmental impositions on society at large (and, of course, on the unvaccinated). No such impositions can be sold to the public if future “relief” is not somehow promised at the same time. This holds true even if cases are increasing — as when China was forced to abandon Zero Covid once Omicron proved irresistible. In short I believe the Covid vaccines’ arrival in late 2020 dramatically protracted the “lockdown mindset” in recipients, in the media, and in governments. Consider Our World In Data’s “Stringency Index”:
So, my position is basically futilist — “Covid protection tools” work, but are not worth having or using. They in fact subvert the ostensible goal of “getting back to normal.” It would have been better (for me, anyway) if they did not exist.
A more mainstream, less futilist perspective, regarding such conclusions as I have just voiced, would probably reply with a more balanced recommendation: Sheltering, masks, and Covid vaccines are probably appropriate for high-risk individuals — the elderly, the already-unwell. No — this still leads to the problem of justifying government impositions.
And, a more mainstream “Covid skeptic” perspective simply insists that the interventions “do not work.” I think this nihilistic impulse is driven by an intuitive, but suppressed understanding of the “futilist” view I have advanced here: From the point of view of individual and social flourishing, of “getting back to normal,” it would be better if they didn’t work (just as I say it would be better if they did not exist). But no one (besides me) is going to say that we should act as if they didn’t even though they do — to acknowledge that masks and Covid vaccines work, but still insist they should not be legal.
Health tradeoffs
Note that I haven’t said anything, above, regarding “health tradeoffs.”
If the health tradeoff is negative, it might be cited as an argument why “a group of people should not take the Covid vaccines” — that is, this group experiences more negative outcomes from vaccination than are reduced due to severe efficacy.
I tend to support such arguments with reservations. It will probably be true that healthy, younger people experience net harms — given that severe efficacy itself is a rare benefit in groups for whom severe disease rarely happens (in children this “benefit” may as well not exist, and doesn’t).
Among my reservations are the fact that for groups where the health tradeoffs are positive, some individuals will still be harmed by the vaccines who would have been fine otherwise; and the above considerations on enabling fear and lockdowns.
Boosters
Severe efficacy is not an argument for mRNA vaccination in general (as explained above) nor for boosters — even on the grounds of reducing severe outcomes. The only “justification” for boosters is putting off first-time infections for as long as possible, which is stupid. Claims of “booster severe efficacy” typically just launder short-term restorations of infection efficacy as an illusory benefit for severe outcomes (there is no or only a trivial improvement to per-infection severe outcome rates, and these improvements are too small to distinguish from whatever biases drive the decision to get a booster).
Finally, the evidence
There is a lot of it. Only a four-part sample will be presented to the reader.
Defining severe efficacy: hosp. per inf.
Severe efficacy is defined here as reducing severe outcomes (chiefly, the disease called “Covid 19”) when one is infected for the first time.
This is distinct from merely “putting off infection to later.” Certainly much of the latter took place in 2021. But Covid vaccinated people were being infected, and so, in many places and contexts, simple comparisons could be made between how they fared after infection vs. the unvaccinated of similar age. This sets aside the bias question until the next section.
i. The Pfizer trial: 1/4 - 1/2 hospitalization per-infection
It is often claimed that the Covid vaccine trials were not “designed” to measure reductions in severe disease. This merely means that they were not all 10 times bigger than they were; not that if they were 10 times bigger, they would have shown, magically, an opposite result.
In trial lingo, severe efficacy was one arm of the “descriptive analysis.” This is hardly a denigrative term: The Prizer trial, in fact, generated a description of severe efficacy. If it had on the other hand described vaccine enhancement (more severe disease), no one would quibble that this safety signal wasn’t what the trial was “designed” to show. The description of severe outcomes is valuable and valid information.
In the “updated” analysis for the Pfizer vaccine (cutoff on March 13, 2021) severe rates and confirmed infection rates any time after dose 1 and 7 days after dose 2 were as follows:

Because the updated analysis cuts off when infection efficacy is only just beginning to wane, infection rates for the vaccinated are about 1/10 of the unvaccinated (the vaccines are still “going strong” at “putting off infections until later”). But severe outcomes (by the FDA definition, which requires hospitalization plus some respiratory stats) are not simply a proxy for “temporarily delayed infections” — rates are 1/30 to 1/21 of the unvaccinated.
I prefer to measure results from the first dose when possible. At all events the “lowering” of the per-infection severe efficacy ratio that results from measuring only after dose 2 simple consists of discounting 9 unvaccinated outcomes for being too “early.” Also, the trial counts 0 vs. 32 post-dose-2 severe outcomes when using the CDC definition of mere hospitalization (which is more translatable to real-world studies).
Of course, one can always dismiss the trial results as Fraud™. It’s a free country.
The point is that, in fact, one is required to play the Fraud™ Card in order to sustain the claim that severe efficacy was never demonstrated (or “described”) in a randomized, blinded context.
Now to move on to a pair of real-world examples.
ii. Israel dashboard: ~2/5 ongoing per infection
The Israel Ministry of Health reported infections and hospitalizations in “real-time” in late 2021. This provided a window into a population with reliable data on vaccination status, but a high likelihood of bias in the choice not to get a vaccine. However, multiple changes were made to the categorization of the vaccinated after boosters were authorized at the end of July — reflecting in general the chaos boosters have created for the problem of tracking efficacy. Rather useful nonetheless was the creation of a category for double-dosed, over 6 months, with no prior infections, which operated in December (before Omicron arrived).
Raw numbers can only be estimated “by eyeball.” Still, the general trend in both age groups is clear — light green and blue are close together in infections, very different for ongoing hospitalizations:
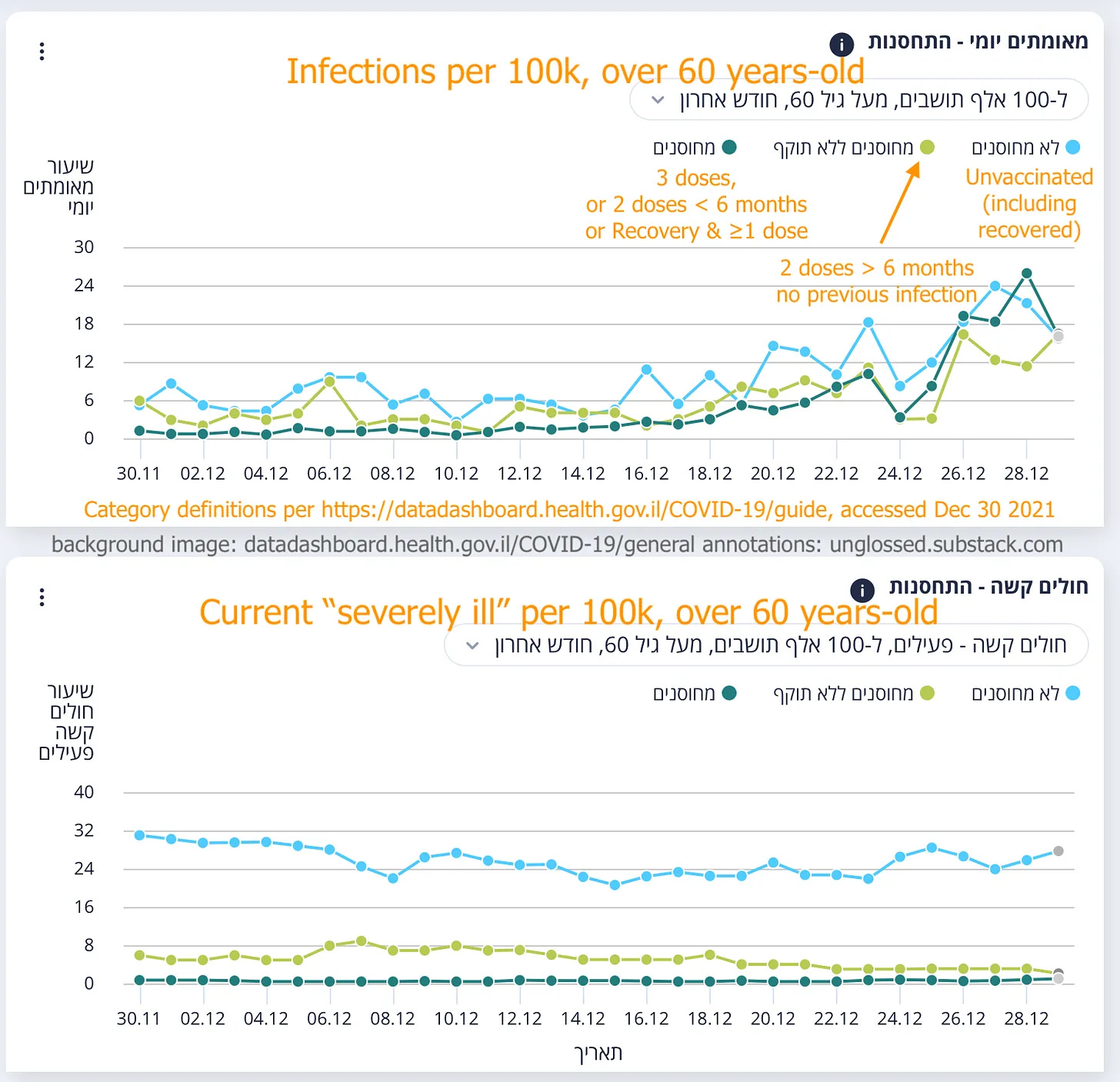
If I try to pin these trends fairly, using a lag for severe outcomes:
iii. England: 1/5 hospitalization per infection (adjusted for age)
Nyberg, et al. sifts through outcomes during the transition to Omicron in England, from November 29 to January 9 of 2021-22.4 In that time, many people are infected; most people at this point have three vaccines, some of them still have no vaccines or still only have two. Among the infected, some go on to be admitted to the hospital — all of this is in the UKHSA.
The authors use this data to produce values of per-infection efficacy against hospitalization according to time since the second (or other) vaccine. This allows for a look at the durability of (“true”) severe efficacy. (Another look, also from England, is here.)
This an important point: At the time, UKHSA data likely had unreliable denominators for the unvaccinated, resulting in widespread reports of “negative infection efficacy.” Whatever the relevance of this problem for the top-line data and for understanding overall outcomes, it doesn’t come into play when considering only those who are reported as infected to begin with — exactly this many people are our denominator.
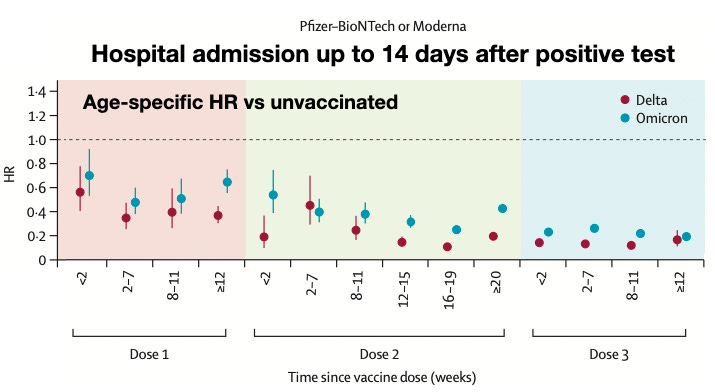
The UK data, however, is probably the most biased in terms of differences between the vaccinated and unvaccinated, especially in older adults (discussed below). For this reason, it would be nice to have raw data on the per-age outcome rates (theoretically one could hack something together from the UKHSA reports). Because the unvaccinated represent less than 10% of older adults, there may be extreme skews at play. Further discussion is in the section on vaccinee bias, below.
iv. US: Vaccine skeptic anecdotes
A survey conducted by Steve Kirsch, consisting of reports by his readers which were intended to reveal patterns in deaths among the vaccinated, shows a substantially higher increase in unvaccinated contact deaths during the Delta and Omicron waves (compared to the relative increase in the vaccinated contacts) — see my previous review. In short, even vaccine skeptics noticed the unvaccinated dying more than the vaccinated in the Delta wave.
Objection 1: Statistical Manipulation
There have been myriad claims regarding statistical manipulation. Outside of reasonable complaints (such as the uselessness of test-negative studies), these claims are unconvincing generally and specifically.
Generally, the replication of severe efficacy findings in multiple regions and contexts renders manipulation implausible as the root explanation for these findings.
Specifically, all claims tend to fall flat. In the instance of the Pfizer trial, just to give an example of the general trend, much has been made of the multitude of “suspected Covid” cases which were not included in the efficacy calculations — even though almost all of these were ruled out by negative PCR tests.
Objection 2: Healthy vaccinee bias
It may be the case that “overall,” the Covid vaccinated are healthier (in some regions or age groups). This is not sufficient to account for observations on the order of 1/5 to 1/2 severe outcomes, per infection or per person, among the vaccinated vs. the unvaccinated.
To take the problem one step at a time:
i. Background does not support “healthy” vaccinee default
To summarize the findings of Remschmidt, et al.5 (copying from their own table):
ii. A lot of unhealthy people clearly got Covid vaccines
Regardless of “overall” health rates, it is obvious that a lot of unhealthy people got Covid vaccines — simply from the photographs of vaccine clinics which flooded the stage in 2021 and afterward.
Here, without accreditation, are the first 9 depictions of active vaccination which appear in a google image search for “Covid vaccine clinic”:
The only unambiguously healthy recipients are the two men in what appear to be staged government photos (or it may be the same man in both).
The inclusion of unhealthy individuals among the Covid vaccinated means that relative infection outcomes among vaccinated and unvaccinated (in the case that the vaccine did nothing) would not be the same as a comparison of “healthy” vs. “unhealthy” groups. Whatever the difference in outcomes is, would be numerically diluted by mixing the two groups together.
iii. “Unhealthiness” doesn’t account for big differences severe outcome rates
Gao, et al. look at the relationship between body-mass index and severe outcomes in England during the spring, 2020 wave.6 They find the following:
As a group, people with extreme obesity (40+) are hospitalized at around 2.5 times the rate of the group with the lowest rate (which is the definition of “health” we are investigating in this context). This is among the most extreme of ratios for pre-existing conditions besides age, being in the neighborhood of cardiovascular disease or hypertension.
And yet in Nyberg, et al., the unvaccinated are hospitalized at five times the rate of individuals 20 weeks past their second dose (per Delta infection).
If the reduction of risk for the Covid vaccinated in fact surpasses undiluted healthy/unhealthy hazard ratios, it cannot be explained by those same ratios. At best, one could speculate that vaccinee bias skews the observed efficacy toward an exaggerated value (as opposed to skewing it toward a lower value), but this still leaves intact the conclusion that severe efficacy is real.
iv. Sub-problem: Uk extreme skews
One complicating factor when it comes to the UK data was the avoidance of vaccinating individuals in hospice and end-of-life-care. This led to short-term skews in overall mortality which far exceeded normal healthy/unhealthy outcome ratios. Additionally, the unvaccinated made up such a small percentage of older age groups that they may in fact look like an “ideal” unhealthy population.
For example, “all-cause” mortality among unvaccinated elderly UK adults in December, 2021, is more than double the vaccinated in each age group in the ONS dataset (which has more reliable denominators than the UKHSA).7 “Double mortality,” however, still leaves the unvaccinated elderly in the “neighborhood” of the severely obese when compared to everyone else. Further, this “double” mortality only corresponded to a few hundred unvaccinated deaths in the same month in each age group — many of which would, in fact, have been driven by severe Covid-19; therefore the “double” mortality cannot simultaneously account for the higher rate of severe Covid-19.
Additionally, there is a limit to the relevance of overall death rate in predicting outcomes of SARS-CoV-2 infection among the elderly. If 95% of UK elderly were vaccinated in December, 2021, then almost all the elderly who were “unhealthy” by any definition would have been vaccinated. Again, the risk ratio from infection without any consideration of the vaccine should have been below 1.5 — something like the difference between “extremely obese” and the nearest group.
v. Summary of the math
If only the extremely obese were unvaccinated in the example above, they would experience outcomes at 2.5 times the rate of the healthiest group. However, since no one else is unvaccinated, the ratio between all vaccinated and the unvaccinated (extremely obese) would not be 2.5, but something between 1 and 2 (say 1.67). Likewise, if only the healthiest group were vaccinated, not all of the unvaccinated would be extremely obese, and the ratio again would be between 1 and 2 (again, say 1.67).
And if on top of all that, a minority of people from all groups made opposite choices of these ideal divisions, the ratio should be expected to fall far below 1.5 (whether in favor of the vaccines or not).
The highest possible values for “efficacy by bias” would seem to be between 9.1% (for a 1.1 outcome ratio) to 28.6% (for a 1.4 outcome ratio). I do not consider the latter value realistic for groups that are stratified by age.
The values for per-infection efficacy observed in the Pfizer trial were 50 - 100%; in the Israel dashboard example, 60% (roughly estimated); in the UKHSA-based study, 25 (first dose) - 80+%.
Vaccinee bias simply cannot possibly account for this. (This can be contrasted with the extremely narrow per-infection “improvements” delivered by boosters, as already acknowledged in the booster section above.)
Objection 3: Biological arguments
This topic I have already addressed, here.
If you derived value from this post, please drop a few coins in your fact-barista’s tip jar.
I have never worn helmets.
I always wear seatbelts. Though these, too, can worsen outcomes in some niche situations (it is not always optimal to remain with the car throughout the entire course of an accident).
Also, ICUs.
Nyberg, T. et al. “Comparative analysis of the risks of hospitalisation and death associated with SARS-CoV-2 omicron (B.1.1.529) and delta (B.1.617.2) variants in England: a cohort study.” Lancet. 2022 Apr 2; 399(10332): 1303–1312.
Remschmidt, C. Wichmann, O. Harder, T. “Frequency and impact of confounding by indication and healthy vaccinee bias in observational studies assessing influenza vaccine effectiveness: a systematic review.” BMC Infect Dis. 2015; 15: 429.
Gao, M. et al. “Associations between body-mass index and COVID-19 severity in 6·9 million people in England: a prospective, community-based, cohort study.” Lancet Diabetes Endocrinol. 2021 Jun;9(6):350-359.
I have this month highlighted in my own import of the ONS data (with the sexes reversed as they were in the ONS publication at the time): https://docs.google.com/spreadsheets/d/1dJNR3XXwaiA9HZVjXxaeP-VH4Lgq7teY3tmpLZlAMBU/edit?usp=sharing
I don’t doubt the efficacy in older adults. I do appreciate your input and well written post.
It is the negative efficacy in younger adults and the SAEs that people died from. I’ve had over $500k in medical bills with injury to my heart lung and eyes. I will not take another vaccine again.
Great article, I applaud the detail level of research that has gone into it.
However. I feel that like many others unfortunately you've fallen into a very common trap, and been tricked into accepting the narrow retrogade perspective of the vaccine fanatical public heath experts, allowing them to set the terms of debate accordingly.
I personally would rate the vaxx as only having a modest and very temporary efficacy. Why? Because real severe efficacy for Covid outcomes comes from having a young healthy immune system, and consider this; the durability of such an immune system is acceptable for around 50 /years/. And it requires no expensive experimental medical intervention with potentially disasterous side effects either.
How can this fraud have been perpetuated successfully on so many people, some of very considerable intelligence? But it's interesting that much of the vaccine critical side has fallen squarely for this trick into merely opposing the assertions of the vaccine fanatics, and so implicitly accepting their terms.