The Bad Biobank Cardiac Study, Briefly
More fodder for exaggerating cardiac impacts from the virus.
Another study amps up the post-infection harms fog machine. Most outcomes seem simply to be reflecting a “with, not from” effect.
Earlier this year, I gave a glowing review to the “Biobank brain change” study.1 The UK Biobank is an ambitious project to track “normal adults” through time, assessing them proactively and periodically for health markers. In that case, brain scans and cognitive performance tests were compared between participants who had or had not been recorded as having a positive test for SARS-CoV-2. The positive-tested fared much worse; as if they had aged extra years compared to the not-positive-tested.
Another new study has been published using Biobank, but this one is, simply, garbage.2
The flaws of this study are simple, regardless of whether the valence, but not extent of the conclusion — infection causes some degree of cardiac harm — is true.
These authors are just trawling event codes in Biobank to fabricate a case / control comparison. This throws out the advantages of Biobank compared to any other database trawl especially as regards a non-biased assessment of health outcomes after the event of interest.3
Namely, because the authors aren’t working with any of the active monitoring assets in Biobank, there is no protection against a “with, not from” effect — participants who show up for cardiac issues and are screened with a PCR test. So, that’s an obvious potential bias for the very health outcomes being measured.
Because it will be asked
Although the timeframe of the study (March 2020 - March 2021) covers the Covid vaccine roll-out, no vaccination status information is provided for analysis.
So with those flaws, everything is poisoned from the start. The positive-test-havers (“Covid-19”) have worse heart health than the Biobank population as a whole (because of the study design, everyone in Biobank is now over 51 years old).
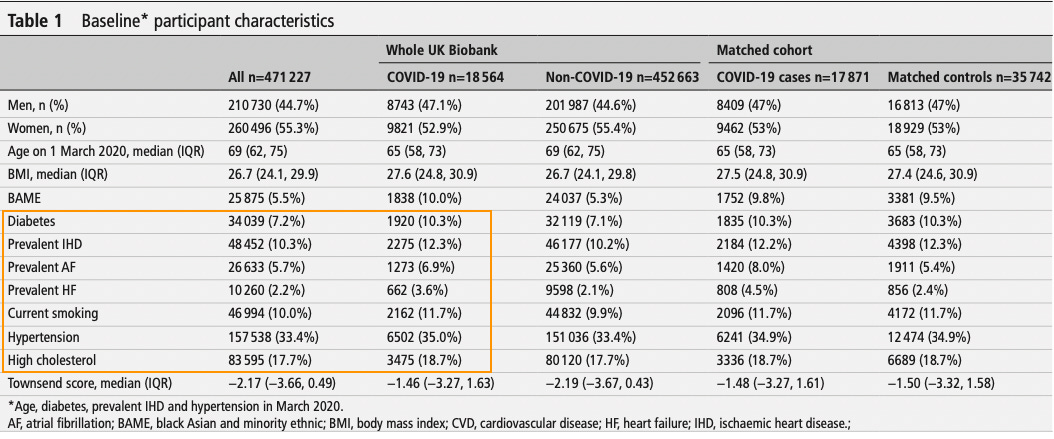
Naturally, the authors “adjust” for these baseline comorbidities by assigning two matched controls from the Non-Covid-19 group. Essentially, for every positive test in a Covid-19 group member, a sticky dart is thrown at the geographically closest two people with the same characteristics in the non Covid-19 group. And when this has been done for all the positive tests, the number of cardiac event codes that happen after tests / darts is counted up and compared. How does that remove the problem of some of those positive tests being caused by cardiac events that were already under way, due to screening upon admission? (It doesn’t.)
The time to event histogram strongly suggests that screening tests upon admission may have driven hits in the Covid-19 group.
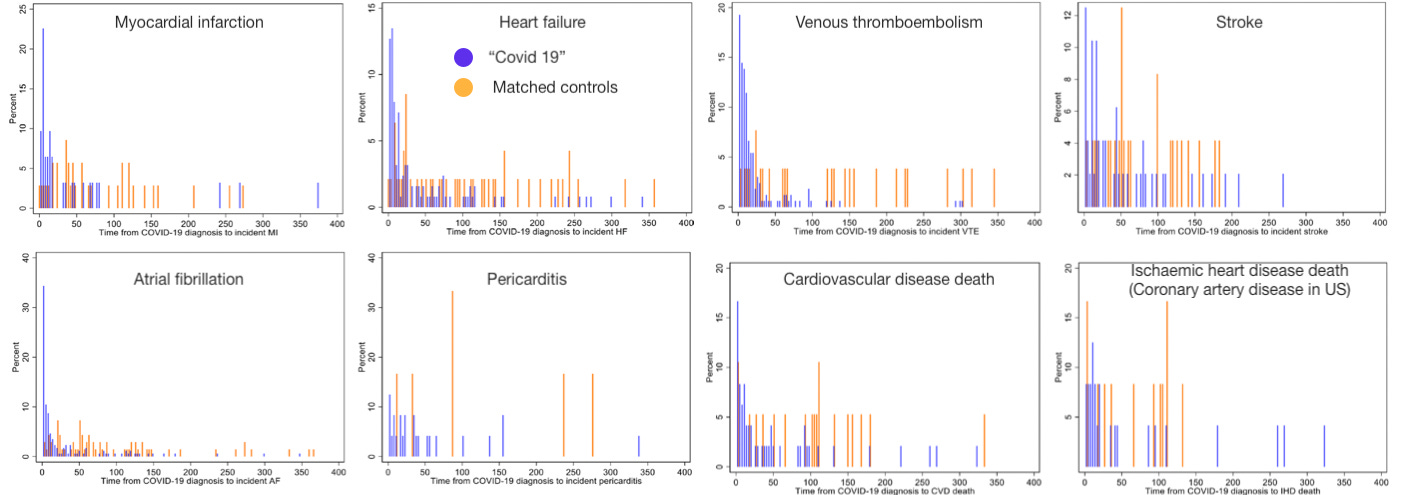
A spot check on atrial fibrillation or myocardial infarction suffice to raise red flags for the entire study design. Does a positive test for for SARS-CoV-2 cause diagnosis of acute AF on Day 0, and then protect from it in a heart-unhealthy population for hundreds of days afterward? (Note that bars do not correspond to equal absolute event counts between both groups, but zero is zero). Does it likewise cause MI in a short span of days, and protect afterward — with lower overall cases? Or are our cases simply people who showed up to an overworked health system with AF or the precursor to MI (i.e. a downturn in health) and were subjected to a test which happened to be positive. The authors comment:
In our main analysis, we found an unexpected association of COVID-19 with lower risk of incident MI in the non- hospitalised subset. It is likely that this finding is a result of selection bias. Individuals who develop mild COVID-19 in the community, but have an MI very soon after would be admitted to hospital and have COVID-19 recorded as a secondary diagnosis. This means that within the non-hospitalised cases we only count events that occur sufficiently separate from the onset of infection, for COVID-19 to not be recorded as a hospital diagnosis. Whereas for their controls, we count events occurring at any time. In effect, the controls have greater time at risk.
I don’t find the logic about different risk times impressive; this does not explain why the histogram back-loads, rather than front-loads protection (note that I am simplifying the distinction between hospitalized and non-hospitalized, which are not separated in the histograms; by definition, the non-hospitalized still continue not to code for AF or MI after days 50 to 80 whereas matched controls do).
So, bearing in mind that the orange bars are also subjected to screening tests, but by definition receive a negative, all that this histogram is showing is some people who showed up for care for atrial fibrillation, some meaninglessly plotted to the day they showed up, some meaninglessly plotted to the day a random neighbor got a positive test, and maybe some that actually experienced this condition in causal connection to a recent positive test and infection.
Because of the elderly, but not-that-elderly nature of our Biobank participants, there would not be much other random screening compared to say, young adults or those of truly advanced age; and the remote-ification of non-emergency healthcare in the UK would ensure that screening for medical care was biased to emergencies. So we have both a signal of extreme bias in the data, and a readily available reason not to be surprised by it. The authors should have thrown this design into the trash and tried something else.
Of course, they didn’t. Tellingly, they also don’t (as far as I can tell) provide any outcome controls — to demonstrate that expected non-cardiac symptoms are associated with a positive test, and that medical codes that shouldn’t have anything to do with infection aren’t.4 It seems a safe bet that no such proofs are reported because they would have blown up the entire study.
That leads us, at last, to the headline results — which only reinforce the obvious red flags we have already observed. Positive tests for SARS-CoV-2 are wildly heart-hazardous if you are hospitalized (because of with, not from screening tests); weirdly heart-protective or neutral if you aren’t hospitalized (likely because the comorbidity matching resulted in an inappropriately un-heart-healthy control group to compare with non-hospitalized infected); and, for unclear reasons highly deadly regardless of hospitalization for infection (there is no all-cause histogram to help figure that one out; and causes of death don’t seem to be listed anywhere; I’ll leave it as a not-interesting-enough mystery).
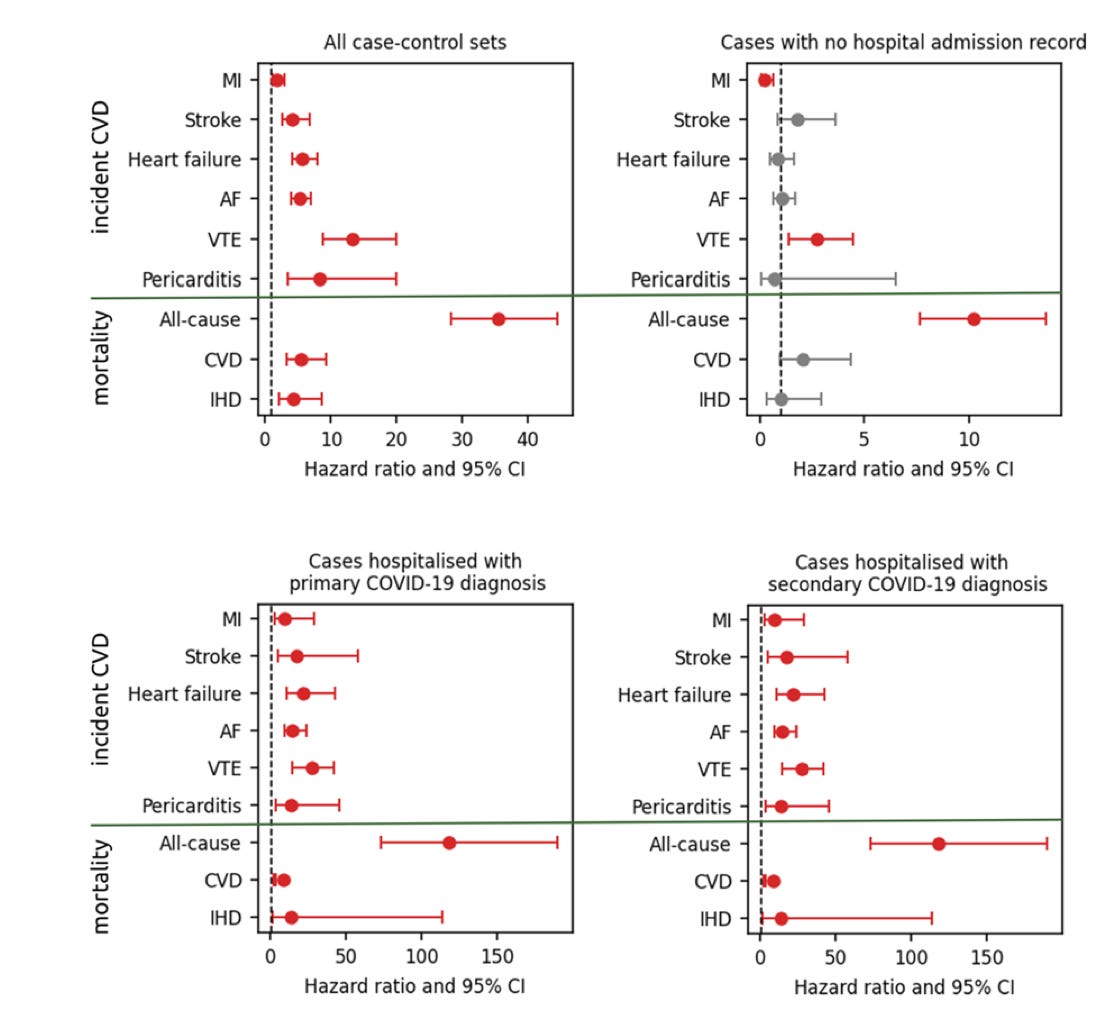
I only hope I have spent as little of the reader’s time possible to convey what an absolute waste of the reader’s time this study represents.
If you derived value from this post, please drop a few coins in your fact-barista’s tip jar.
Raisi-Estabragh, Z. et al. “Cardiovascular disease and mortality sequelae of COVID-19 in the UK Biobank.” Heart Published Online First: 24 October 2022.
Biobank, “the most detailed, long-term prospective health research study in the world,” is described in the post linked in footnote 1 and at https://www.ukbiobank.ac.uk/learn-more-about-uk-biobank/about-us
This compares to the Veteran’s Affairs database series, where the negative outcome controls always seem cherry-picked. I intend a review of that series to summarize what it does and does not seem to tell us.
Jeez, having to wade through the junk studies is unfortunate. Thanks for the assist. 👍🏽💕
I took part in the Biobank epidemiology study as believed in their plan to find new treatments.
Every 5 years you had an MOT with them.
Roll on scamdemic. I woke up.
I got a phone call asking why I didn’t have my jab, when I asked who was calling, the phone call was cut off. But they knew my DoB and post code, hmm. I called Gp and asked had they tried to call me, they denied calling me. Who had my info, and who knew I hadn’t took my jab, hmm
Roll on a year, and as I investigated the scamdemic and the reasons why they were culling us, I came across Jeremy Farrar, which led to the Wellcome Trust, which ran Biobank. Ahhh, ding, that’s who called me. What did they have in store for me