Frequently, Unglossed devotes posts to clarifying why certain lines of attack against the experimental spike protein transfections, aka the “injectable bioweapons,” aka the Covid vaccines, are not valid.
This is not to “defend” the Covid vaccines as an end in of itself, but to save the reader time spent reading (future) and recycling (past) faulty content.
In this case, I am not sure if my take of the data is 100%. I am a bit confused at just what “the data” being allegedly presented is.
However, I think I can make a strong case that the reader is provisionally better off not taking the post in question as valid.
So, this was posted, by the blogger who goes by “el gato malo.”
Typically when someone hereabouts in “Covid vaccine skeptical Substack” wades into the ONS spreadsheets, they commit some error in interpreting the data, and then I either ignore their work or post a guide to the error as a sort of community service announcement.
(“Experimental gene therapy injections probably bad; however, ONS data still doesn’t have any smoking guns” is essentially the official Unglossed line in this department.)
Apologies for that two-part egocentric prelude — I just want to make it clear that my interest is simply in sparing the reader faulty analysis; regardless of whether that faulty analysis supports what I believe to be true.
In this case I simply do not know what to make of things. Egm appears to be fishing through the quite-old, but latest-available ONS spreadsheet to piece together a supposedly “clean” all cause mortality comparison by Covid-vaccine-injection status (any / none).
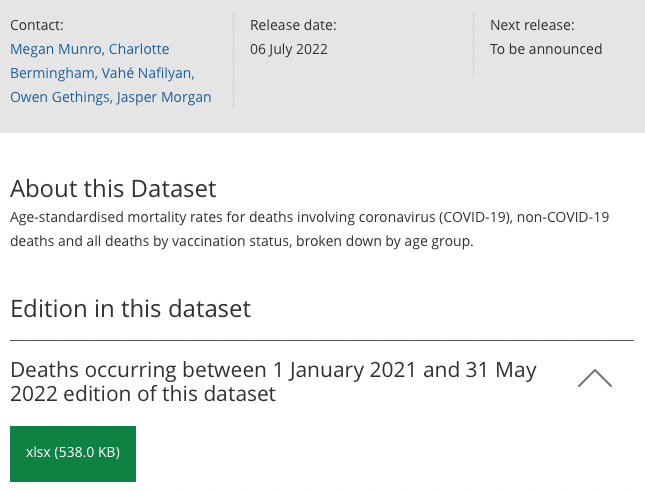
Citing the opacity of other ways the ONS treats this data (their age-standardized calculations) as his warrant for raiding the ONS spreadsheet for easter eggs, egm proceeds to produce… a completely opaque dataset analysis.
He cites a NIMS/ONS graph as the source for his by-age any / none (Covid vaccine) denominators —
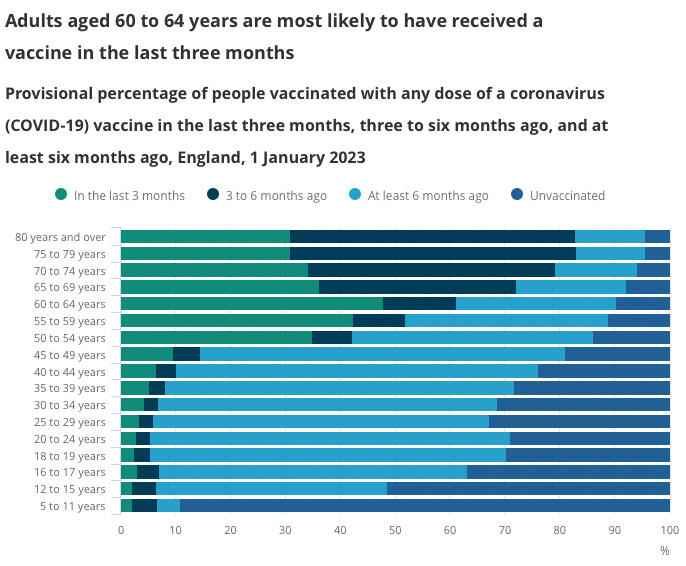
— And then plots what (as far as I can tell) should be the result of
(any monthly deaths / (any monthly deaths + none monthly deaths))/any injection percent of population on the UKHSA-NIMS graph.
*any = any injection, or “ever vaccinated”; none = unvaccinated
Just which ONS table is egm using for deaths? Have I simply missed where this is specified? There are different tables with two different death counts.
Here I have partially compiled table 2 by-age death counts for January, 2022 (partial because there is a formatting issue in table 2 that is preventing easy math-ing of the death values):
The reason for this discrepancy is presumably that the ONS sheets pull data from multiple sources.
I think the smaller set of numbers is limited to individuals who are in all three of the sources for the Public Health Data Asset, based on the read-me for an older version of the data.
Data sources
ASMRs are created using the Public Health Data Asset (PHDA), a linked dataset combining the 2011 Census, the General Practice Extraction Service (GPES) data for coronavirus (COVID-19) pandemic planning and research, and the Hospital Episode Statistics (HES). We linked vaccination data from the National Immunisation Management Service (NIMS) to the PHDA based on NHS number.
Source: ons.gov.uk
Which might imply that the Table 8 values use fewer filters. Either way, what the reader should be aware of right off the bat is that the smaller numbers are indexed to clearly provided person-years at risk. Table 2 thus offers monthly all-cause mortality values where the correct denominators are expressly given.
Why no one has “noticed” the ONS “smoking gun” before (because it doesn’t show higher mortality):
Imagine an example “January”:
The person-years fill up as people spend days being in a given status. If a person spends 3 days unvaccinated and then gets injected, 3 of their days go in the never injected bucket. Deaths refer to status at the time of death. You divide the top square by the bottom square for each group.
Although the ONS uses a granular, time-since-X-dose format for this portion, it is trivial in theory to construct a person-year-rated, overall “any vaccine” by-age-group death rate from these sheets; producing iron-clad evidence of a negative all cause mortality effect from injection.
So why hasn’t anyone in Covid vaccine skeptical substack done so?
Because aside from the very young (where healthy user bias might be flipped), the ONS 3-contact data from Table 2 shows lower all-cause mortality in the injected.
Previous misfires involving the ONS have used the “time since dose” data-artifacts to suggest that the Covid-vaccinated “die after 6 months” — ignoring the fact that Covid-vaccinated continue to be Covid-vaccinated when they get additional injections. When you put all the categories together, the mortality ends up being lower (except in the very young). This has resulted in at least a few cases of slinking away from bold claims, once the bucket problem was perceived.
What is novel about today’s (apparent) misfire is that it takes on the all-injected data and somehow flips the result.
Since the formatting is being buggy (I have to reenter death values to make the formula see them in Google Sheets); I merely spot-checked 80 year-olds in January and April of 2022.
What egm shows:
What table 2 shows:
In both January and April, the ever-injected are dying at a lower rate per person-year than the unvaccinated. This is likely due to the extreme healthy user bias that prevails in England (given that the unvaccinated were also dying at a higher rate all throughout 2021, so the ever-vaccinated are here in catch-up mode against a year of pull-forward effect, and yet are still better off).
Importantly, the issue with Table 8 (if that is what egm has used) is not that the rates of deaths are flipped. Rather, table 8 simply liberates egm from using the ONS’s own person-year values as the denominator — so all that is happening is that the unvaccinated denominator becomes almost twice as large.
With that said, it’s still not exactly clear to me just how egm gets to where he ends up, math-wise. If I use “4%” for the 80-89 unvaccinated rate (the graph lists the 80 and above unvaccinated rate as 4%); I still get a different result for January, 2022. So if anyone has a clue what happened here, please advise in the comments.
Here are table 8 deaths compared to table 2 person-years (which makes the unvaccinated rate look even worse, as Table 8 adds more deaths in this group proportionately) and to the UKHSA graph denominator (which almost flips the rates; but still doesn’t get to egm’s 1.03 ratio (162.68/174.75 = .93):
Argument against using the UKHSA graph as the denominator
If the ONS’s own person-year values show lower all cause mortality (per person-year) in the ever-injected vs. the unvaccinated, then that is what should be taken as representing the mortality rates for the individuals in that data-set.
In the first place, it is not reasonable to fish around outside the data set for a different denominator that flips the results. One might as well be up-front and invent random “true denominators” based on “gut feeling.”
Second, the use of overlapping contact points in the ONS data set almost certainly minimizes overestimation of the unvaccinated denominator — the set primarily counts individuals who are still “in” the system (whether it includes the three overlapping contact points that I noted above, or a different filter), whereas UKHSA/NIMS might count individuals who haven’t been in the country for a while.
In which case voila,1 magic immortal “unvaccinated” people:
(any monthly deaths / (any monthly deaths + none monthly deaths [missing deaths that occur outside of the system due to having moved, etc.]))/any injection percent of population on the UKHSA-NIMS graph [which is not as high as in real life].
You layer two depressed denominators; ensuring an inflated risk ratio. It isn’t rocket science.
If you derived value from this post, please drop a few coins in your fact-barista’s tip jar.
The original version of this post used “viola,” as I frequently forget that actually loudly saying “viola” in English does not reflect the correct spelling.
And here I’m going to type an extra sentence just to try to fix a substack drafts conflict bug that is driving me crazy.
And here another. Just ignore. Technical difficulties.
Ok now I will hit publish.
As someone who wrote several posts on mortality, I must note something that we should all be aware of.
It is not directly related to this Brian's post but I thought I can express my feelings here.
ANALYZING MORTALITY IN-DEPTH IS VERY DIFFICULT.
They invented randomized trials partly to bypass these difficulties.
For example, let's say that a fat, triple boosted guy named Bob dies a month after having his third COVID.
Having this person in mortality statistics, and having his demographic characteristics, can we answer a simple question: what killed Bob? Fatness? Covid? Vaccines? Cholesterol? Or something else?
Some people allege a healthy user bias. Some people allege unhealthy user bias. etc etc
It is not very easy. It is also not very easy to do causality properly on the population level.
This is why most respectable nations have demographics institutes, full of statisticians, MDs etc etc.
I could, for example, do a regression of "deaths" by "boosters", note a positive relationship and write about "association". Yeah, there is an association.
But how much proof do we have? I tried hard to find "proof" beyond correlations and sometimes, I believe I found other convincing statistical things, like having similar slopes in UK deprivation quartiles and worldwide country level statistics. That increases our strong suspicions about vaccines.
But proof "beyond reasonable doubt" may remain elusive. So many confounders can be alleged.
We can only prove something by analyzing individual level data on millions of people, and hopefully doing some targeted interventions.
The data is not available, the authorities are ignoring excess mortality and discontinuing their reports.
This leaves us wondering, naturally, if something nefarious is going on.
And something nefarious IS going on because ignoring excess mortality IS nefarious by definition.
I dunno why you are wrestling with these probably faulty data-sets when Ron Unz tells us that the problem is Obesity!
https://www.unz.com/runz/obesity-and-the-end-of-the-vaxxing-debate/
I guess the reason I am not dead yet despite being unvaxxed is that I am not obese. After all, the fake President told me that as an unvaxxed person I was going to die last winter or and the latest this winter (although, this winter is not over yet.)
Maybe he skipped over the bit on his teleprompter where it said "you fat fucks are gonna die this winter if you are not vaxxed!"
It would be good to know.