Trollish Study Challenge / Poll
Follow up to the Cleveland Clinic "boosters increase infections" study.
A week ago (insanely; the holidays have certainly killed my productivity) I shot out a brief post regarding the already-infamous Cleveland Clinic Study purporting to associate increased doses with increased infection.
As I pointed out, this was merely an artifact of how “catching up with infections” would look in a “real-time” view, without taking overall (“lifetime”) infections into account.
Like most of what I write, my argument has had no influence on the groupthink opinion about the study. But I submit to the reader that the groupthink opinion is simply a product of widespread “statistical illusion illiteracy” — most people, including those like me who anoint themselves as outsider science journalists, simply cannot come to grips with how merely assigning categories to people creates distortions and bias all the time.
As a follow-up exercise to illustrate this point, I submit the following weekend poll.
Which of the groups of Cleveland Clinic workers in this graph from Shrestha, et al., has experienced the most infections in the last six months (July to December, 2022)?
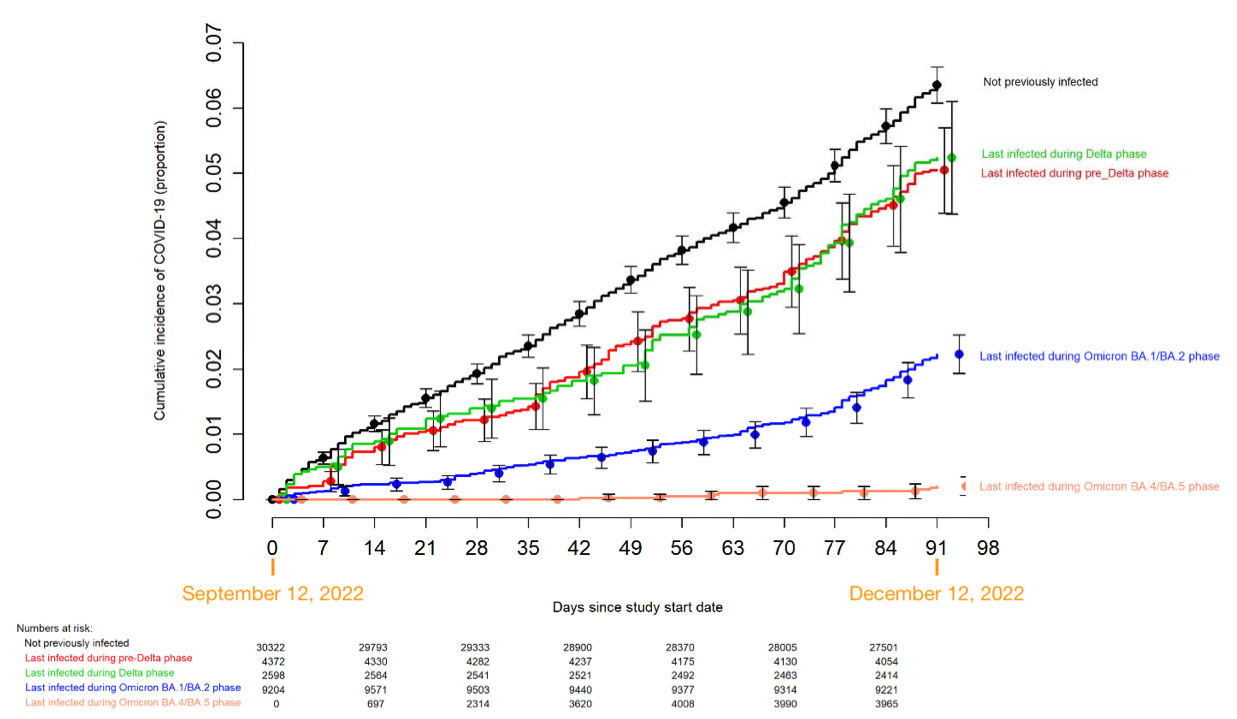
Why a poll? Just to engage the reader with a tactile option-selecting experience. Scroll below for the answer and comments.
Cheat-Notes:
To save the reader from wasting time in the study text, here is the makeup of the groups in the plot above, even though it gives away the answer to the challenge:
As an additional note, subjects are censored for 90 days after a positive test (so that additional positives do not count as an “infection” until after the 90 day window, and meanwhile, subjects are not in the “at risk” pool). And, Table 3 “proximate exposure” windows are calculated separately and are not relevant to the categories in Fig 1.
Answer
Naturally, the correct answer in rhetorical quizzes is always whatever is “counter-intuitive.”
In this case, the 4,087 individuals in the BA.4/5 group [the flat orange line] experienced the most infections in the last 6 months (4,087 vs. some fraction of 2,452 for each of the other groups).
Although Shrestha, et al. are not precise with their time cutoffs for the variant definitions, we can be sure that all 4,087 of the BA.4/5 group were infected after mid-June because they do not begin to become “at risk” again until a week after September 12; after they have left the 90-day window since their positive test.
OK, but is there actually a point to this exercise?
Well, let’s take what has been the widespread, groupthink interpretation of the study: The most-previously-injected were the ones who became infected at the highest rate from mid-September to mid-December, “because negative efficacy.”
But if this is generalizable, then it means that they also became infected at the highest rate just before the study begins, from mid-June to mid-September. Which means they are heavily represented in the BA.4/5 group, which experiences the least infections from mid-September to mid-December. Which also means, that in the next three months, the heavily-boosted who were infected during the study period will be the least infected.
A paradox thus arises where the highly boosted are the most likely to be infected in any given current period “x,” and thus also most likely to be in the category of least likely to be infected in the “x+1” period. This paradox is only sustainable if it reflects arbitrarily segmenting a transition toward an equilibrium into discrete “real-time” units.
So all that the study is showing is that the highly-boosted are still catching up with natural immunity.
If you derived value from this post, please drop a few coins in your fact-barista’s tip jar.
Very interesting.
Data can be presented and interpreted and re-interpreted and over interpreted. The details and parameters are often skimmed over and missed. The devil is in the detail. It helps to be cynical and nit picky. Pedanticism only exists as a concept for those who don't appreciate the details and subtleties therin. People so often simply see what they want to see and remain happy in their self imposed echo chamber.
My bottom line is that we have a personal responsibility to think for ourselves. To be as healthy as we can be - by ourselves and for our own self. Then the whole data thing is interesting when viewed as an outsider. I have come to realise that the data we are given is not just open to interpretation, it is massively open to fraud. We are presented all these figures and charts and numbers and statistics which we have to take at face value. But what of them are actually real or as they initially appear? Governments all over the world have stopped publishing and/or updating data. Data accumulated in sites such as ourworldindata take information as given by the governments. So - what is real, what is hidden, unknown, unassessed and misinterpreted?
Who knows. I don't. Even when I think I know I still keep questioning. The answers will either confirm or enlighten... So sad that so many people grow out of the 'why?' phase of life at such an early stage.
Whoops, a bit of a ramble there.
Thanks for posting this Brian, it's good work.
"But if this is generalizable, then it means that they also became infected at the highest rate just before the study begins, from mid-June to mid-September. Which means they are heavily represented in the BA.4/5 group, which experiences the least infections from mid-September to mid-December. Which also means, that in the next three months, the heavily-boosted who were infected during the study period will be the least infected."
I don't think so. Fall infection rates were about 2% in the no-dose group, 3.3% in the 1-dose group, 4.6% in the 2-dose group, 5.5% in the 3 dose group and 6% in the > 3 dose group. All are well under 10% and vary by at most a factor of 3. There were more infections in the summer. If we assume the ratios between groups didn't vary that tells us rates were about 3% in the no-dosers and 10% in the >3 dosers. Removing 10% of the >3 dosers from the eligibility pool (or even the probability of infection pool) due to recent infection still leaves 90% to get infected in the fall. It's a simple matter to see that the same stable infection rates could be maintained from season to season due to low-enough infection rates to minimize the number of recently infected and therefore more immune.
The same paper reports an bivalent booster efficacy of 30%. They are not at all clear about the reference point for the bivalent RR calculation. Using the already elevated rates for 2, 3 or 3+ doses is a great way to cheat. But let's just assume 30% to be generous. Is this relative to the That suggests a window of perhaps 60 to at most 120 days of some degree of vaccine efficacy in preventing at least a detected infection. This suggests that perhaps there was still summer efficacy for some of the >3 dosers who were vaccinated in the spring, but probably 1-3 months earlier relative to the risk window start date than the bivalent boosted were for the fall. So perhaps there is a bit of catch up, but I would argue most of this is higher infection rates in those with lots of doses, especially among those with no dose within about the past 90 days.